Home
Multidimensional Stationary Time Series: Dimension Reduction and Prediction
Barnes and Noble
Multidimensional Stationary Time Series: Dimension Reduction and Prediction
Current price: $180.00
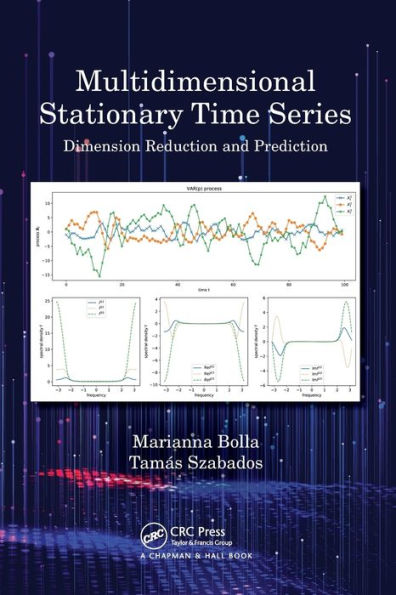
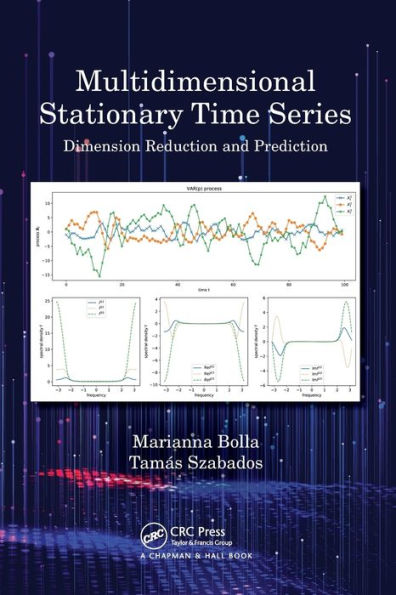
Barnes and Noble
Multidimensional Stationary Time Series: Dimension Reduction and Prediction
Current price: $180.00
Size: Hardcover
Loading Inventory...
*Product information may vary - to confirm product availability, pricing, shipping and return information please contact Barnes and Noble
This book gives a brief survey of the theory of multidimensional (multivariate), weakly stationary time series, with emphasis on dimension reduction and prediction. Understanding the covered material requires a certain mathematical maturity, a degree of knowledge in probability theory, linear algebra, and also in real, complex and functional analysis. For this, the cited literature and the Appendix contain all necessary material. The main tools of the book include harmonic analysis, some abstract algebra, and state space methods: linear time-invariant filters, factorization of rational spectral densities, and methods that reduce the rank of the spectral density matrix.
Serves to find analogies between classical results (Cramer, Wold, Kolmogorov, Wiener, Kálmán, Rozanov) and up-to-date methods for dimension reduction in multidimensional time series
Provides a unified treatment for time and frequency domain inferences by using machinery of complex and harmonic analysis, spectral and SmithMcMillan decompositions. Establishes analogies between the time and frequency domain notions and calculations
Discusses the Wold's decomposition and the Kolmogorov's classification together, by distinguishing between different types of singularities. Understanding the remote past helps us to characterize the ideal situation where there is a regular part at present. Examples and constructions are also given
Establishes a common outline structure for the state space models, prediction, and innovation algorithms with unified notions and principles, which is applicable to real-life high frequency time series
It is an ideal companion for graduate students studying the theory of multivariate time series and researchers working in this field.
Serves to find analogies between classical results (Cramer, Wold, Kolmogorov, Wiener, Kálmán, Rozanov) and up-to-date methods for dimension reduction in multidimensional time series
Provides a unified treatment for time and frequency domain inferences by using machinery of complex and harmonic analysis, spectral and SmithMcMillan decompositions. Establishes analogies between the time and frequency domain notions and calculations
Discusses the Wold's decomposition and the Kolmogorov's classification together, by distinguishing between different types of singularities. Understanding the remote past helps us to characterize the ideal situation where there is a regular part at present. Examples and constructions are also given
Establishes a common outline structure for the state space models, prediction, and innovation algorithms with unified notions and principles, which is applicable to real-life high frequency time series
It is an ideal companion for graduate students studying the theory of multivariate time series and researchers working in this field.