Home
On the Fracture Behaviour and the Fracture Pattern Morphology of Tempered Soda-Lime Glass
Barnes and Noble
On the Fracture Behaviour and the Fracture Pattern Morphology of Tempered Soda-Lime Glass
Current price: $54.99
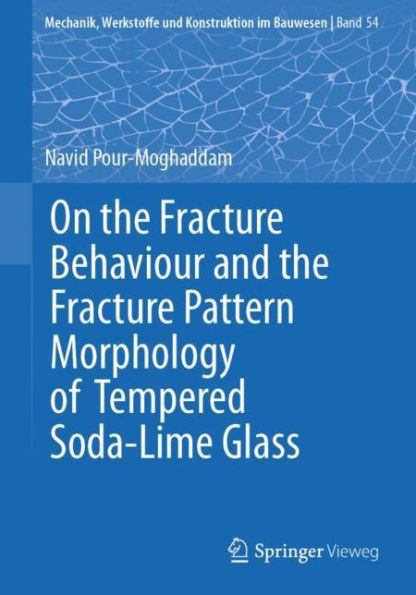
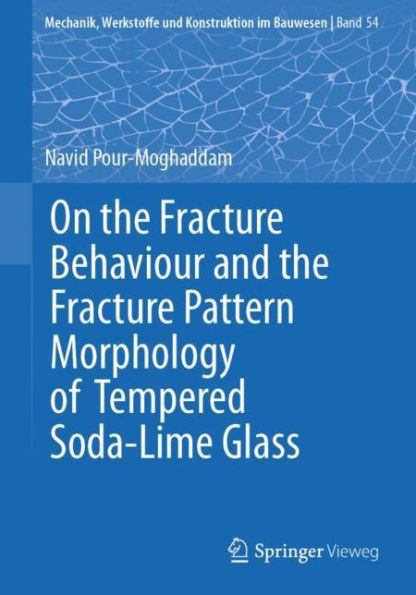
Barnes and Noble
On the Fracture Behaviour and the Fracture Pattern Morphology of Tempered Soda-Lime Glass
Current price: $54.99
Size: OS
Loading Inventory...
*Product information may vary - to confirm product availability, pricing, shipping and return information please contact Barnes and Noble
The main criteria for assessing the load-bearing behaviour and risk potential of monolithic glass are its fragmentation and the morphology of the fragments. These depend strongly non-linearly on the strain energy density present in the glass at the time of fracture, which can be converted into fracture energy. Thus, the design and optimization of structural glazing in engineering requires both knowledge of the relevant parameters and mechanisms during the fracture process in glass and an understanding of the characteristics of the fracture structure. Based on fracture mechanics considerations and comprehensive experimental investigations, various aspects and physical quantities of fracture behaviour as well as characteristics and parameters of fracture pattern morphology of fragmented, tempered soda-lime glass were studied and correlated with the stored strain energy. The relationship between fragmentation behavior and strain energy was elaborated using the energy criterion in Linear Elastic Fracture Mechanics (LEFM) related to the initial strain energy before fragmentation and in the post-fracture state. Furthermore, a machine learning inspired approach for the prediction of 2D macro-scale fragmentation of tempered glass was developed and elaborated based on fracture mechanics considerations and statistical analysis of the fracture pattern morphology. A method was deduced and applied in which the fracture pattern of tempered glass is predicted and simulated by Voronoi tessellation of point patterns based on Bayesian spatial point statistics fed with energy conditions in LEFM.