Home
Reproducible Econometrics Using R
Barnes and Noble
Reproducible Econometrics Using R
Current price: $74.00
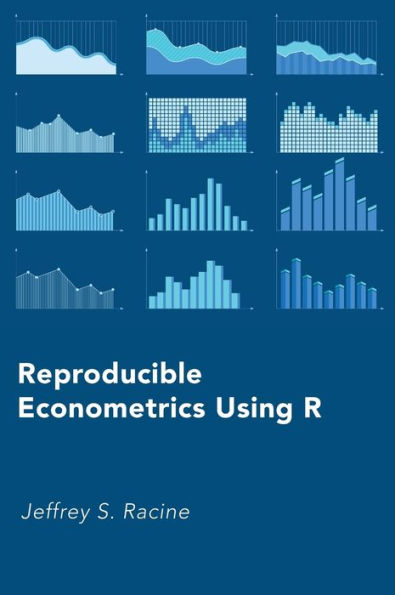
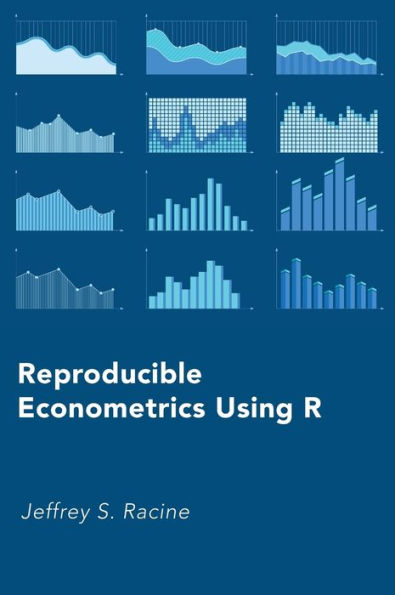
Barnes and Noble
Reproducible Econometrics Using R
Current price: $74.00
Size: Hardcover
Loading Inventory...
*Product information may vary - to confirm product availability, pricing, shipping and return information please contact Barnes and Noble
Across the social sciences there has been increasing focus on reproducibility, i.e., the ability to examine a study's data and methods to ensure accuracy by reproducing the study.
combines an overview of key issues and methods with an introduction to how to use them using open source software (R) and recently developed tools (R Markdown and bookdown) that allow the reader to engage in reproducible econometric research.
Jeffrey S. Racine provides a step-by-step approach, and covers five sets of topics, i) linear time series models, ii) robust inference, iii) robust estimation, iv) model uncertainty, and v) advanced topics. The time series material highlights the difference between time-series analysis, which focuses on forecasting, versus cross-sectional analysis, where the focus is typically on model parameters that have economic interpretations. For the time series material, the reader begins with a discussion of random walks, white noise, and non-stationarity. The reader is next exposed to the pitfalls of using standard inferential procedures that are popular in cross sectional settings when modelling time series data, and is introduced to alternative procedures that form the basis for linear time series analysis. For the robust inference material, the reader is introduced to the potential advantages of bootstrapping and the Jackknifing versus the use of asymptotic theory, and a range of numerical approaches are presented. For the robust estimation material, the reader is presented with a discussion of issues surrounding outliers in data and methods for addressing their presence. Finally, the model uncertainly material outlines two dominant approaches for dealing with model uncertainty, namely model selection and model averaging.
Throughout the book there is an emphasis on the benefits of using R and other open source tools for ensuring reproducibility. The advanced material covers machine learning methods (support vector machines that are useful for classification) and nonparametric kernel regression which provides the reader with more advanced methods for confronting model uncertainty. The book is well suited for advanced undergraduate and graduate students alike. Assignments, exams, slides, and a solution manual are available for instructors.