Home
Rule-Based Evolutionary Online Learning Systems: A Principled Approach to LCS Analysis and Design / Edition 1
Barnes and Noble
Rule-Based Evolutionary Online Learning Systems: A Principled Approach to LCS Analysis and Design / Edition 1
Current price: $109.99
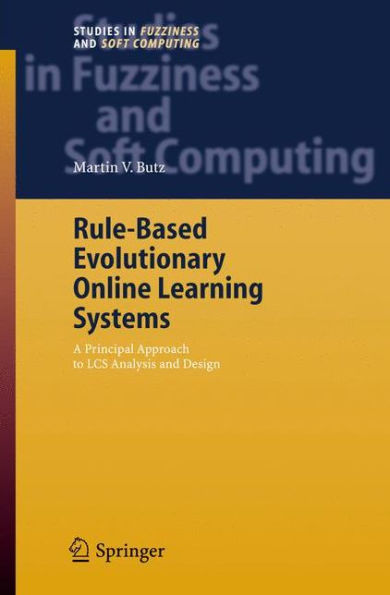
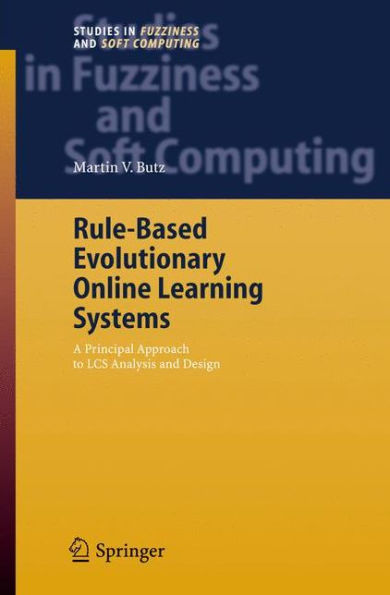
Barnes and Noble
Rule-Based Evolutionary Online Learning Systems: A Principled Approach to LCS Analysis and Design / Edition 1
Current price: $109.99
Size: OS
Loading Inventory...
*Product information may vary - to confirm product availability, pricing, shipping and return information please contact Barnes and Noble
Rule-based evolutionary online learning systems, of ten referred to as Michig- style learning classifier systems (LCSs), were proposed nearly thirty years ago (Holland, 1976; Holland, 1977) originally calling them cognitive systems. LCSs combine the strength of reinforcement learning with the generali- tion capabilities of genetic algorithms promising aexible, online general- ing, solely reinforcement dependent learning system. However, despite several initial successful applications of LCSs and their interesting relations with a- mal learning and cognition, understanding of the systems remained somewhat obscured. Questions concerning learning complexity or convergence remained unanswered. Performance in different problem types, problem structures, c- ceptspaces, and hypo thesis spaces stayed nearly unpredictable. Thisbookhas the following three major objectives: (1) to establish a facetwise theory - proach for LCSs that promotes system analysis, understanding, and design; (2) to analyze, evaluate, and enhance the XCS classifier system (Wilson, 1995) by the means of the facetwise approach establishing a fundamental XCS learning theory; (3) to identify both the major advantages of an LCS-based learning approach as well as the most promising potential application areas. Achieving these three objectives leads to a rigorous understanding of LCS functioning that enables the successful application of LCSs to diverse problem types and problem domains. The quantitative analysis of XCS shows that the inter- tive, evolutionary-based online learning mechanism works machine learning competitively yielding a low-order polynomial learning complexity. Moreover, the facetwise analysis approach facilitates the successful design of more - vanced LCSs including Holland’s originally envisioned cognitivesystems. Martin V.